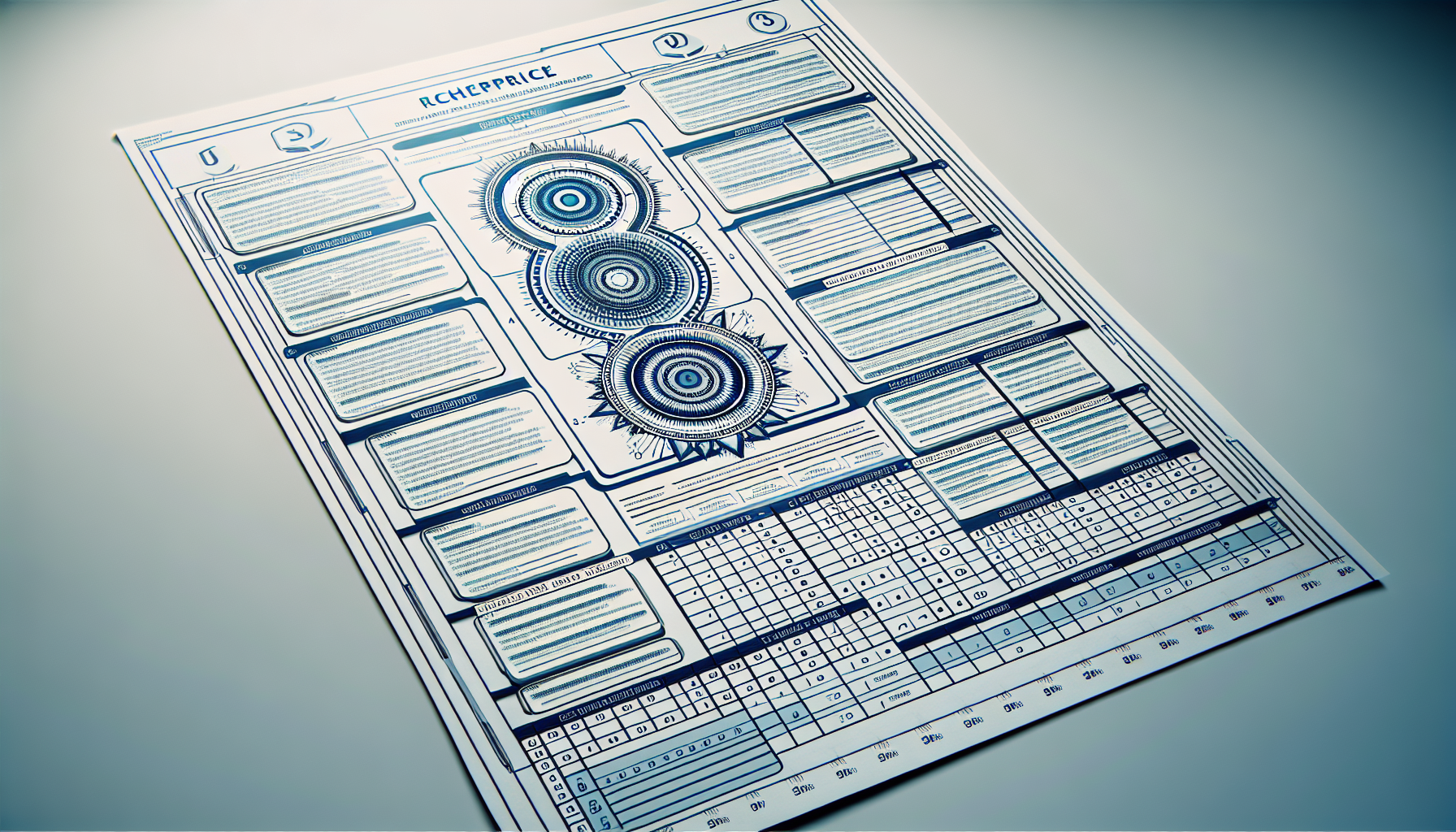
About Course
Module 5: Advanced Rubric Design
Overview:
In this module, we will explore advanced techniques for designing rubrics tailored to specific AI applications. We will also delve into dynamic and adaptive rubrics that evolve based on continuous feedback, ensuring that evaluation criteria remain relevant and effective.
Topic 9: Customizing Rubrics for Specific AI Applications
Lesson 25: Rubrics for Natural Language Processing (NLP)
Evaluating NLP models requires rubrics that address the unique challenges of language understanding and generation. Key considerations include:
Language Understanding: Assessing how well the model comprehends context, semantics, and syntax.
researchgate.net
+1
planitteachers.ai
+1
Language Generation: Evaluating the fluency, coherence, and relevance of the text produced by the model.
teacherspayteachers.com
Task-Specific Metrics: Incorporating metrics like BLEU, ROUGE, and METEOR for tasks such as translation and summarization.
Lesson 26: Rubrics for Computer Vision
Computer vision models interpret visual data, necessitating rubrics that focus on:
researchgate.net
Accuracy: Measuring the model’s precision in tasks like image classification and object detection.
Robustness: Evaluating performance across varying conditions, such as different lighting or angles.
psu.edu
+4
teacherspayteachers.com
+4
researchgate.net
+4
Efficiency: Considering processing speed and resource utilization.
Lesson 27: Rubrics for Reinforcement Learning (RL)
RL models learn through interactions with their environment. Effective rubrics should assess:
Learning Efficiency: The speed at which the model learns optimal behaviors.
Adaptability: The ability to generalize learned behaviors to new, unseen scenarios.
researchgate.net
Stability: Consistency in performance without erratic behaviors.
Topic 10: Dynamic and Adaptive Rubrics
Lesson 28: Introduction to Dynamic Rubrics
Dynamic rubrics are designed to evolve in response to new data and insights. They adapt to changing requirements, ensuring continuous alignment with learning objectives.
asccc.org
+2
planitteachers.ai
+2
researchgate.net
+2
Lesson 29: Creating Adaptive Evaluation Criteria
Adaptive rubrics adjust their criteria based on ongoing performance data. This approach allows for personalized assessments that cater to individual learning trajectories.
planitteachers.ai
essaygrader.ai
+2
getmagicbox.com
+2
planitteachers.ai
+2
Lesson 30: Implementing Feedback Loops
Incorporating feedback loops into rubric design ensures continuous improvement. By systematically collecting and analyzing feedback, rubrics can be refined to better meet evaluation goals.
ibbaka.com
Quiz 5: Advanced Rubric Design
To conclude this module, a quiz will assess your understanding of advanced rubric design principles, customization for specific AI applications, and the implementation of dynamic and adaptive rubrics.
Course Content
Topic 9: Customizing Rubrics for Specific AI Applications
-
Lesson 26: Rubrics for Computer Vision
-
Lesson 27: Rubrics for Reinforcement Learning